Quickstart
Hespi takes images of specimen sheets from herbaria and first detects the various components of the sheet.
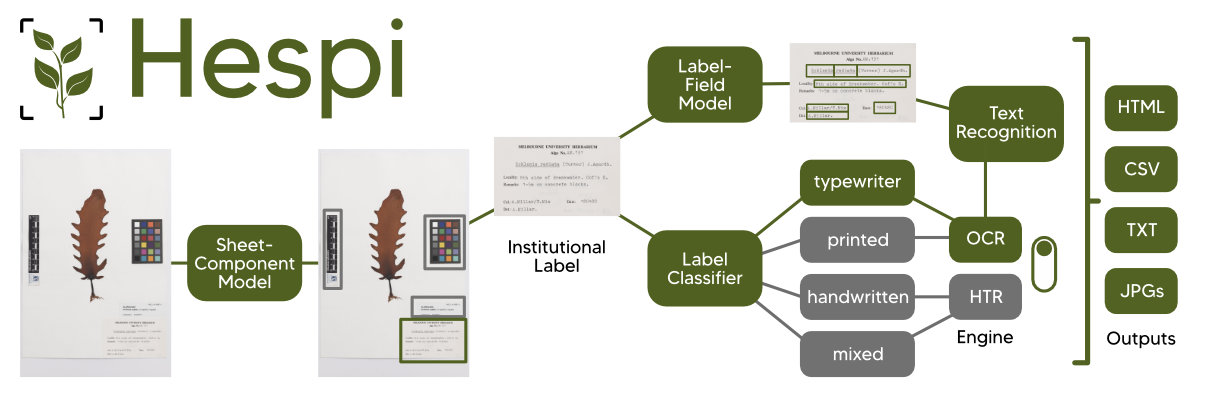
Hespi first takes a specimen sheet and detects the various components of it using the Sheet-Component Model. Then any full database label detected is cropped and this is given to the Label-Field Model which detects different textual fields written on the label. A Label Classifier is also used to determine the type of text written on the label. If it is printed or typewritten, then the text of each field is given to an Optical Character Recognition (OCR) engine and if there is handwriting, then each field is given to the Handwritten Text Recognition (HTR) engine. The recognized text is then corrected using a multimodal Large Language Model (LLM). Finally, the result of the fields is post-processed before being written into an HTML report, a CSV file and text files.
The stages of the pipeline are explained in the documentation for the pipeline.
Installation
Install hespi using pip:
pip install hespi
The first time it runs, it will download the required model weights from the internet.
It is recommended that you also install Tesseract so that this can be used in the text recognition part of the pipeline.
To install the development version, see the documentation for contributing.
Usage
To run the pipeline, use the executable hespi
and give it any number of images:
hespi image1.jpg image2.jpg
By default the output will go to a directory called hespi-output
.
You can set the output directory with the command with the --output-dir
argument:
hespi images/*.tif --output-dir ./hespi-output
The detected components and text fields will be cropped and stored in the output directory.
There will also be a CSV file with the filename hespi-results.csv
in the output directory with the text recognition results for any institutional labels found.
By default hespi
will use OpenAI’s gpt-4o
large language model (LLM) in the pipeline to produce the final results.
If you wish to use a different model from OpenAI or Anthropic, add it on the command-line like this: --llm MODEL_NAME
You will need to include an API key for the LLM. This can be OPENAI_API_KEY
for an OpenAI LLM or ANTHROPIC_API_KEY
for Anthropic.
You can also pass the API key to hespi with the --llm-api-key API_KEY
argument.
More information on the command line arguments can be found in the Command Line Reference in the documentation.
There is another command line utility called hespi-tools
which provides additional functionality.
See the documentation for more information.
Training with custom data
To train the model with custom data, see the documention.